Alternative Dispute Resolution
Feb. 14, 2025
From checkmate to mediating trust cases: AI's next move
Trust mediators must prepare for a future where AI's analytical power could reshape negotiation strategies--while potentially leaving attorneys as passive recipients of intelligence they don't fully understand.




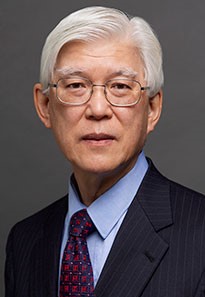
John H. Sugiyama
Arbitrator and Mediator
JAMS
Phone: (415) 774-2617
Email: jsugiyama@jamsadr.com
Hon. John H. Sugiyama (Ret.) is a mediator at JAMS with experience in myriad legal fields and disciplines adjudicating complex matters through trial and alternative dispute resolution processes. Judge Sugiyama presided for 18 years on the Contra Costa County Superior Court. During the last nine years of his judicial career, he served as the supervising judge for the Probate Division of his superior court. He may be reached at jsugiyama@jamsadr.com.
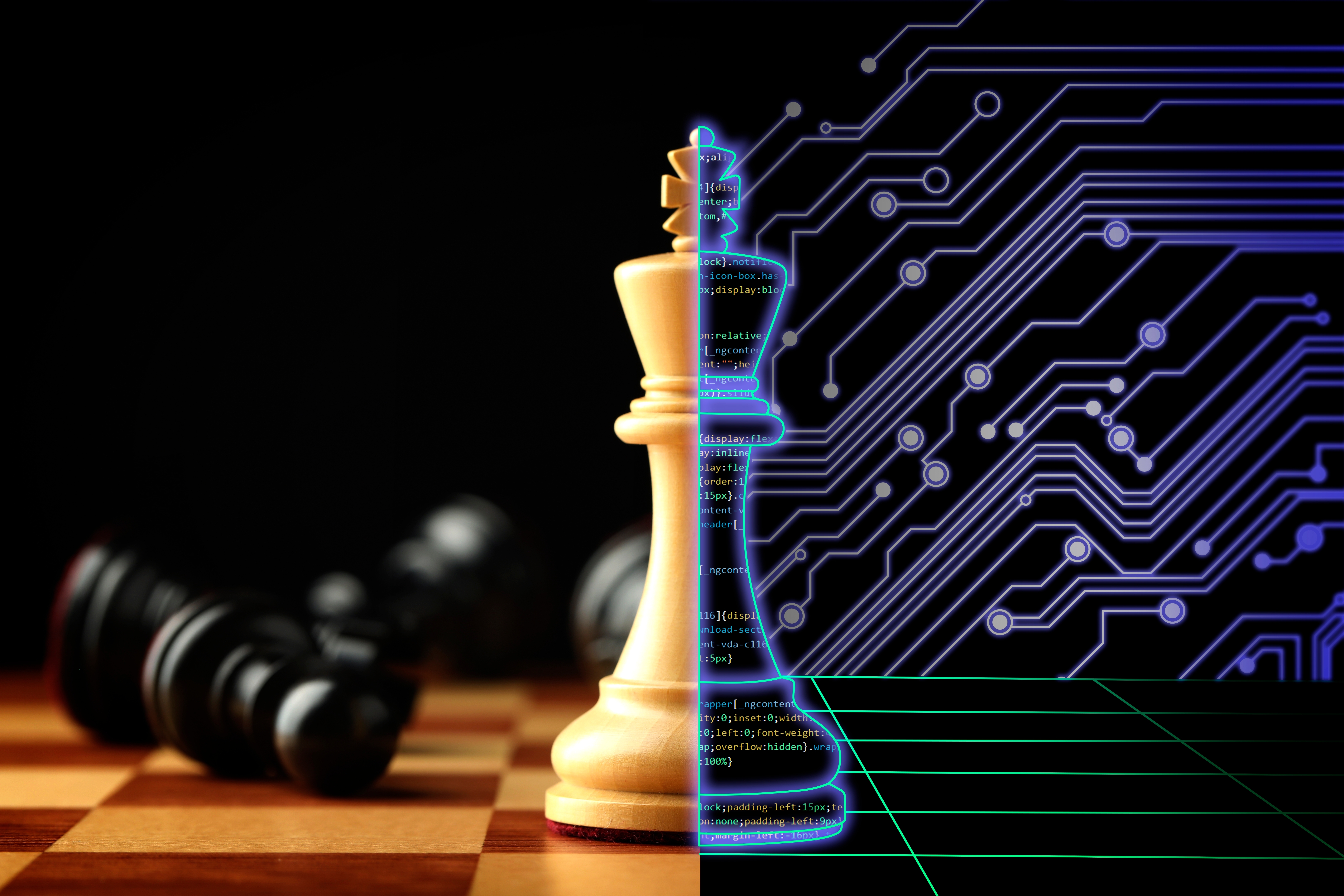
Within a few years, certainly before the end of the decade, attorneys engaged in trust advocacy will use artificial intelligence programs with advanced large language and generative capabilities, enhanced by emergent planning capacity. The programs will principally guide pre-negotiation strategic planning and perhaps secondarily support tactical decision-making during mediations.
When that time comes, attorneys will face the specter of becoming passive, albeit beneficial, recipients of a form of intelligence they will not fully understand. They will not know how AI operates: how it sorts through unimaginably vast bits of data, selects from among them and formulates Delphic pronouncements. They will risk abdicating control without fully grasping that they are doing so. To avoid this relegation, they should endeavor to acquire and retain the ability to obtain both an explanation from AI of how it formulates its pronouncements and the opportunity to obtain a second opinion from AI if the first seems questionable.
In practice, neither explanation nor opportunity will likely be forthcoming. Yet, once adopted by one attorney, all in the technocratic society will come to employ AI. Preceding acceptance, however, certain issues await resolution.
Goal identification
Before the creation of term papers for stressed students and briefs for well-intentioned attorneys, AI entered popular consciousness through its application to the strategy board games of chess and Go. Played universally, the games are appreciated for their seeming complexity. The eventual emergence of AI's superiority in the games occurred over two decades. A brief examination of that progression illuminates some of the challenges of applying AI to trust advocacy.
For the games, the requirements for victory are relatively easy to grasp: the capture of the opposing player's king in chess and the control of more territory than the adversary in Go. In trust mediation, the concept of winning is a distraction.
In elemental terms, the goal in the latter may be conceived as the resolution of an underlying dispute over a trust on terms that are acceptable to the participating parties. But each trust-related dispute is different. What is resolution? What are acceptable terms?
Furthermore, in trust mediation, the goal may be subjective, lacking rational basis. Greed, hubris and fear are all emotions that influence parties. Attorneys seek to account for their existence. Yet their pernicious presence may not become evident until an impasse is reached in negotiations.
To find application in trust mediation, AI programs consequently must be designed to accommodate multiple goals that may be influenced by subjective factors that defy easy description and explanation. Although daunting, accounting for the influence of amorphous emotional impediments nonetheless will not be insurmountable.
Ironically in this context, AI, like Spock of Star Trek, is impervious to emotion. Anxiety, apprehension and insecurity are not embedded in its operating systems. It functions without the restrictive impulses of fear or shame, assimilating only the logical.
AI programs thus build on objectively discernable elements. The critical facet of trust mediation in this respect is that the ascertainment of goals can be done objectively for three interrelated reasons: Settlement terms will usually not exceed those achievable through trial, the determination of probable trial results can be deduced objectively, and the comparison of proposed settlement terms with probable trial results can similarly be accomplished objectively.
In this way, the objectively achievable constrains the subjectively desirable. If a mediation proposal falls within the range of a probable trial result, settlement should follow. Of course, a party could reject seemingly favorable settlement terms for inexplicable reasons. But in most instances, a party will not risk a self-destructive loss of inheritance. As Machiavelli reflected centuries ago, "[M]en more quickly forget the death of their father than the loss of their patrimony."
Program networks
If AI programs for trust mediation can be conceived on a foundation of objectively ascertainable elements, their development will be inevitable because the challenge to mathematicians to create them will be irresistible. The art of negotiation follows game theory. Game theory derives from mathematics. Mathematics imbues the soul of AI.
The progression of AI applications from chess to Go lends further support for this sense of inevitability. In 1997, after a setback the prior year, an IBM supercomputer named Deep Blue defeated then-world chess champion Garry Kasparov in a six-game match, 3 1/2 to 2 1/2. In 2016, AlphaGo, a program developed by Google DeepMind, won four of five games against Lee Sedol, one of the strongest players in the history of Go.
The passage of nearly 20 years between these matches underscores the differences in complexity between chess and Go. Although more kinds of pieces are involved in chess than in Go, the latter is vastly more complex in actual play. In chess, about 400 moves are possible after the first two; in Go, after the first two moves, about 130,000 are possible. In total, more moves may be made in Go than exist atoms in the universe.
During the years from one match to the other, an exponential expansion of computing power accompanied by a corresponding increase in programming ingenuity fueled progression from the brute-force AI employed by Deep Blue to the kind of AI displayed by AlphaGo. In the latter instance, what today are deemed large language and generative capacities were brought together in ways that enabled the program not only to absorb the data to which it had access, but also in effect to learn from internal simulations using that data. Hence, applications of that sort perhaps should more accurately be designated as generative artificial, or GAI, instead of AI alone.
As a remarkable facet of that generative capacity, glimpses of intuitive creativity revealed themselves amid demonstrations of enormous computing power. AlphaGo had strata of big data and search networks, upon which the moves of countless prior games were entered into vast memory banks for replay in subsequent games based on probabilistic calculations by a ferociously fast search algorithm. But AlphaGo also had layered into its networks an algorithm that mimicked human intuition. Indeed, in the second game, which it won, AlphaGo made a move that, as revealed by internal data, had only a one in 10,000 probability of play by any human. That sublime moment, now famously identified simply as Move 37, provided a foreshadowing of creative thought not previously evident in AI.
Thus, facets of intuition and creativity can be built into AI. Move 37 confirms that they will.
Data retrieval
Chess and Go have their own unique notation systems that enable games to be reported for subsequent review by players of any level of proficiency anywhere in the world. Now virtually all games played in any tournament are reported, often instantaneously.
These records, spanning almost two centuries for chess and decades for Go, gave the developers of Deep Blue and AlphaGo access to data encompassing thousands of games. Once entered in memory banks, the data became available for retrieval through robust search networks.
Data about trust litigation may also be recorded, somewhere, but not necessarily accessible in easily transcribable form. The data, however, eventually will be retrieved from petitions for approval of settlement agreement and trial statements of decision.
Settlement agreements themselves will be difficult to access and mine due to the confidentiality with which they are cloaked. Inferences about settlement terms, however, may be gleaned indirectly. After successful mediation, to foreclose later challenges and to obtain judicial oversite for enforcement, attorneys often file petitions for approval of settlement agreements. The petitions may be found in official court records. They usually contain summaries of the matters in dispute. They may also allude to the terms with which the parties are expected to abide.
Trial statements of decision, also filed in official court records, may be another source of relevant data. From their factual findings and judicial conclusions, reasonable deductions can be made about the probable judgment that could be rendered in similar proceedings. These probability determinations can serve to temper wildly inflated expectations that can animate participants in mediations arising in disputes like those that have been decided through trial.
Providers of alternative dispute resolution services may perhaps also be a source of relevant data. Even though their records are confidential and proprietary, they nevertheless have a keen interest in technological developments that affect them. AI developers thus may be able to forge agreements with them to share redacted data that does not reveal privileged information.
Overlay of networks
Eventually and inevitably, as these issues are answered, AI programs will be developed that hold the promise of facilitating the work of attorneys engaged in trust advocacy. The architectural structure of the programs will consist of an overlay of network algorithms designed to perform multiple functions, among which will include ones that:
Identify mediation goals
Analyze probable trial outcomes if settlement is not attained
Organize data about mediations and trials
Calculate fees and costs of mediation and, alternatively, trial
Estimate when a trial may be calendared if settlement is not attained
Mimic intuitive thought about subjective impediments to resolution in trust mediations (derived from large language searches of articles and other materials written by attorneys engaged in related fields)
Provide a search function to retrieve relevant data in a hierarchical order.
When these AI programs emerge, attorneys will find themselves compelled to use them, because unavoidably they will be there. When that occurs, attorneys will be bewildered by the gift they have been given. AI will not explain itself. Nor will AI offer second opinions. Whether attorneys can coexist and even coevolve with AI will dictate where this challenging journey of discovery will lead.
Submit your own column for publication to Diana Bosetti
For reprint rights or to order a copy of your photo:
Email
Jeremy_Ellis@dailyjournal.com
for prices.
Direct dial: 213-229-5424
Send a letter to the editor:
Email: letters@dailyjournal.com